To get the best of both worlds – the sensitivity of LC-MS with the speed of PS-MS – and a functional substrate that can maintain sample integrity, LLNL researchers looked to 3D printing. They have patented a novel approach to create lattice spray substrates for direct ionization mass spectroscopy using 3D-printing processes.
Image
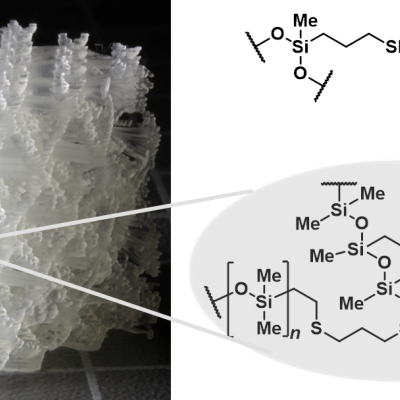
LLNL researchers, through careful control over the chemistry, network formation, and crosslink density of the ink formulations as well as introduction of selected additives, have been successful in preparing 3D printable silicone inks with tunable material properties. For DIW (direct in writing) applications, LLNL has a growing IP portfolio around 3D printable silicone feedstocks for diverse…
Image

LLNL’s method of 3D printing fiber-reinforced composites has two enabling features:
Image
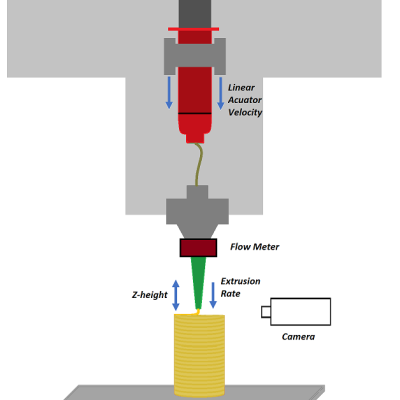
Livermore researchers have developed a method for implementing closed-loop control in extrusion printing processes by means of novel sensing, machine learning, and optimal control algorithms for the optimization of printing parameters and controllability. The system includes a suite of sensors, including cameras, voltage and current meters, scales, etc., that provide in-situ process monitoring…
Image
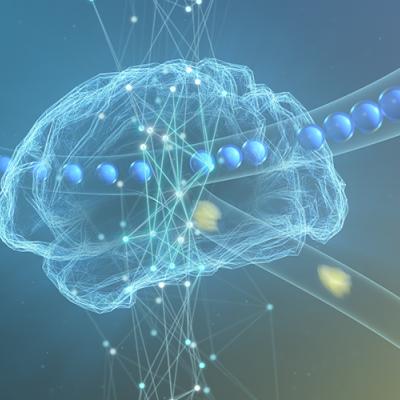
LLNL researchers have developed a system that relies on machine learning to monitor microfluidic devices. The system includes (at least) a microfluidic device, sensor(s), and a local network computer. The system could also include a camera that takes real-time images of channel(s) within an operating microfluidic device. A subset of these images can be used to train/teach a machine learning…