LLNL’s Distributed Implicit Neural Representation (DINR) is a novel approach to 4D time-space reconstruction of dynamic objects. DINR is the first technology to enable 4D imaging of dynamic objects at sufficiently high spatial and temporal resolutions that are necessary for real world medical and industrial applications.
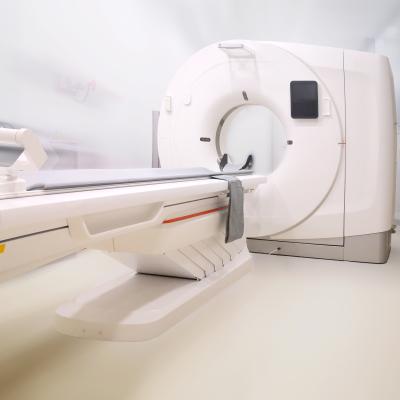
The essence of this invention is a method that couples network architecture using neural implicit representations coupled with a novel parametric motion field to perform limited angle 4D-CT reconstruction of deforming scenes.
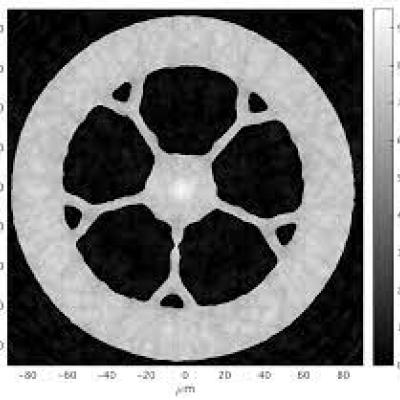
To solve these challenges using new and existing CT system designs, LLNL has developed an innovative software package for CT data processing and reconstruction. Livermore Tomography Tools (LTT) is a modern integrated software package that includes all aspects of CT modeling, simulation, reconstruction, and analysis algorithms based on the latest research in the field. LTT contains the most…
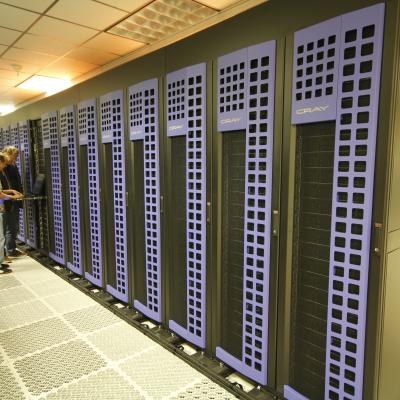
Clinical images have a wealth of data that are currently untapped by physicians and machine learning (ML) methods alike. Most ML methods require more data than is available to sufficiently train them. In order to obtain all data contained in a clinical image, it is imperative to be able to utilize multimodal, or various types of, data such as tags or identifications, especially where spatial…
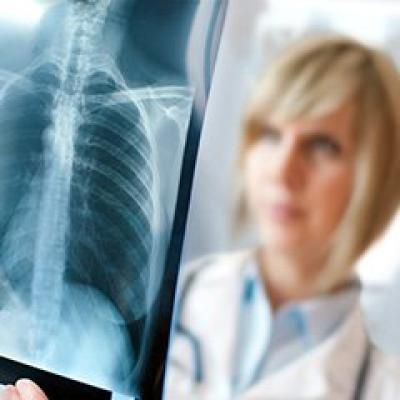
Some COVID-19 diagnoses are utilizing computed tomography (CT)-scans for triage. CT-scans produce immediate results with high sensitivity. The digital images produced by a CT-scan require physicians to identify objects within the image to determine the presence of disease. Object identification can be done using machine learning (ML) techniques such as deep learning (DL) to improve speed and…