Improving the active material of the Zn anode is critical to improving the practicality of Zn-MnO2 battery technology. LLNL researchers have developed a new category of 3D structured Zn anode using a direct-ink writing (DIW) printing process to create innovative hierarchical architectures. The DIW ink, which is a gel-based mixture composed of zinc metal powder and organic binders, is extruded…
Keywords
- Show all (76)
- Additive Manufacturing (37)
- 3D Printing (7)
- Data Science (5)
- Cybersecurity (4)
- Manufacturing Improvements (3)
- Computing (2)
- Imaging Systems (2)
- Synthesis and Processing (2)
- Analysis (1)
- Information Technology (1)
- Manufacturing Simulation (1)
- Material Design (1)
- Microfabrication (1)
- Precision Engineering (1)
- Quantum Science (1)
- Sensors (1)
- Volumetric Additive Manufacturing (1)
- (-) Manufacturing Automation (2)
- (-) Simulation (2)
- (-) Electric Grid (1)
Image
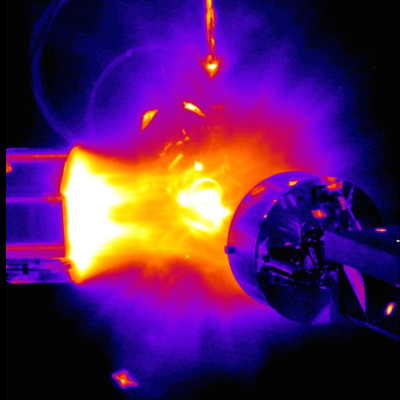
CRETIN is a 1D, 2D, and 3D non-local thermodynamic equilibrium (NLTE) atomic kinetics/radiation transport code which follows the time evolution of atomic populations and photon distributions as radiation interacts with a plasma consisting of an arbitrary mix of elements. It can provide detailed spectra for comparing with experimental diagnostics.
Image
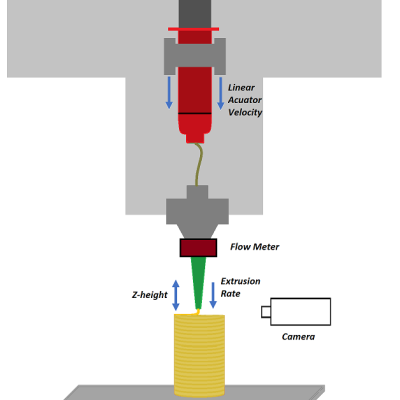
Livermore researchers have developed a method for implementing closed-loop control in extrusion printing processes by means of novel sensing, machine learning, and optimal control algorithms for the optimization of printing parameters and controllability. The system includes a suite of sensors, including cameras, voltage and current meters, scales, etc., that provide in-situ process monitoring…
Image
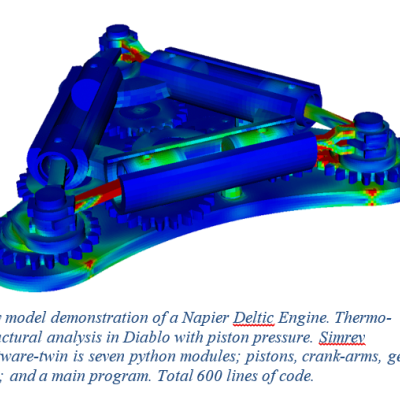
Simrev is a python library imported into a user-generated program. As the program grows in capability and complexity, the engineered product matures. The "software twin" handles all changes to product configuration and is the portal to running supercomputing analysis and managing workflow for engineering simulation codes. Assemblies become program modules; parts, materials, boundary conditions…
Image
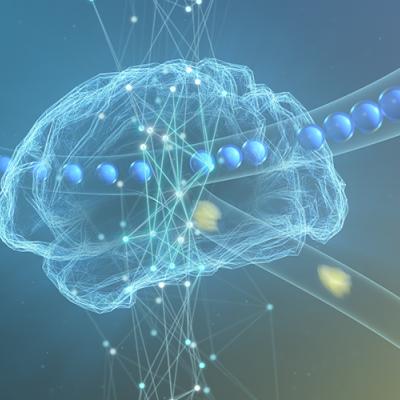
LLNL researchers have developed a system that relies on machine learning to monitor microfluidic devices. The system includes (at least) a microfluidic device, sensor(s), and a local network computer. The system could also include a camera that takes real-time images of channel(s) within an operating microfluidic device. A subset of these images can be used to train/teach a machine learning…