Keywords
- Show all (76)
- Additive Manufacturing (37)
- 3D Printing (7)
- Data Science (5)
- Cybersecurity (4)
- Manufacturing Improvements (3)
- Computing (2)
- Imaging Systems (2)
- Simulation (2)
- Synthesis and Processing (2)
- Analysis (1)
- Electric Grid (1)
- Manufacturing Simulation (1)
- Material Design (1)
- Microfabrication (1)
- Precision Engineering (1)
- Quantum Science (1)
- Sensors (1)
- Volumetric Additive Manufacturing (1)
- (-) Manufacturing Automation (2)
- (-) Information Technology (1)
Technology Portfolios
Image
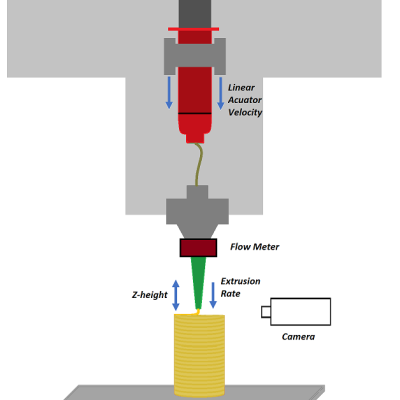
Livermore researchers have developed a method for implementing closed-loop control in extrusion printing processes by means of novel sensing, machine learning, and optimal control algorithms for the optimization of printing parameters and controllability. The system includes a suite of sensors, including cameras, voltage and current meters, scales, etc., that provide in-situ process monitoring…
Image
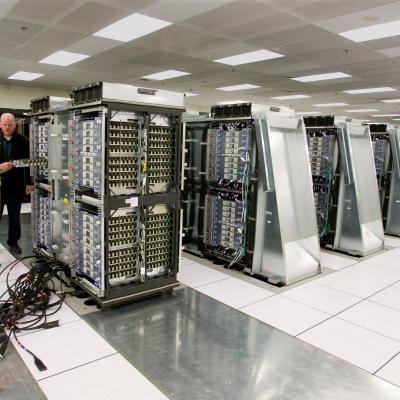
LLNL has developed a new active memory data reorganization engine. In the simplest case, data can be reorganized within the memory system to present a new view of the data. The new view may be a subset or a rearrangement of the original data. As an example, an array of structures might be more efficiently accessed by a CPU as a structure of arrays. Active memory can assemble an alternative…
Image
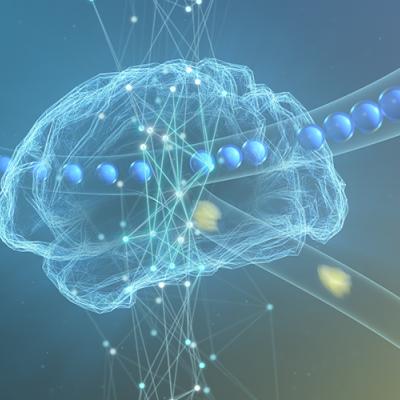
LLNL researchers have developed a system that relies on machine learning to monitor microfluidic devices. The system includes (at least) a microfluidic device, sensor(s), and a local network computer. The system could also include a camera that takes real-time images of channel(s) within an operating microfluidic device. A subset of these images can be used to train/teach a machine learning…