LLNL researchers have developed a self-supporting structural material that promises more efficient carbon capture specifically from air, but generally from all CO2 containing gas sources. The material is produced with a liquid high-amine-content precursor polymer that is functionalized by adding on polymerizable end groups.
Keywords
- Show all (234)
- Additive Manufacturing (53)
- Instrumentation (41)
- Synthesis and Processing (20)
- Sensors (14)
- Diagnostics (12)
- Imaging Systems (9)
- Photoconductive Semiconductor Switches (PCSS) (9)
- 3D Printing (8)
- Electric Grid (7)
- Materials for Energy Products (7)
- Substrate Engraved Meta-Surface (SEMS) (7)
- Therapeutics (7)
- Carbon Utilization (6)
- Semiconductors (6)
- Brain Computer Interface (BCI) (5)
- Compact Space Telescopes (5)
- Optical Switches (5)
- Diode Lasers (4)
- Laser Materials Processing (4)
- (-) Data Science (5)
Technology Portfolios
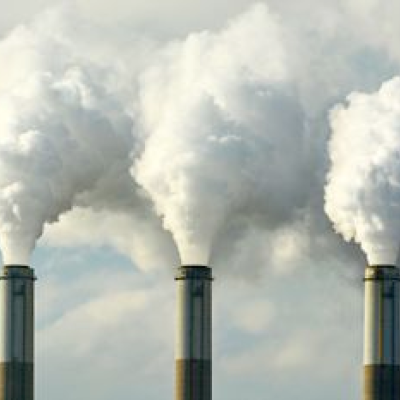
This invention solves a limitation in the current practice of adding hydroxyl functional groups to the aminopolymer through the use of an alternative synthetic approach. The novelty of our approach is to produce new structurally modified relatives of common aminopolymers (PEI and PPI) as well as new functionalized materials in which the hydroxyl groups are tethered to a carbon in the backbone…
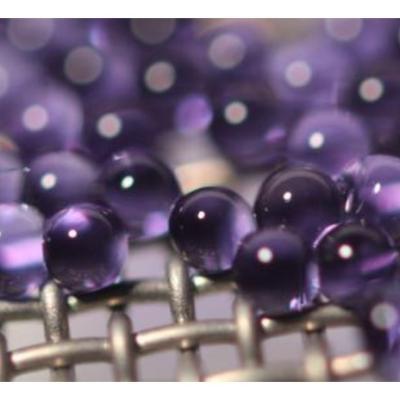
This invention describes a multiple nozzle microfluidic unit that allows simultaneous generation streams of multiple layered coaxial liquid jets. Liquids are pumped into the device at a combined flow rate from 100 mL/hr to 10 L/hr. Droplets are created with diameters in the range of 1 µm to 5 mm and can be created with 1-2 shell layers encapsulating fluid. Droplets created from the system can…
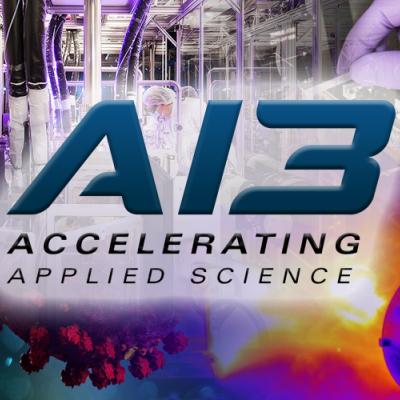
Lawrence Livermore National Laboratory (LLNL) is offering the opportunity to collaborate in accelerating artificial intelligence (AI) for applied science, including research in key areas such as advanced material design, 3D printing, predictive biology, energy systems, “self-driving” lasers and fusion energy research.
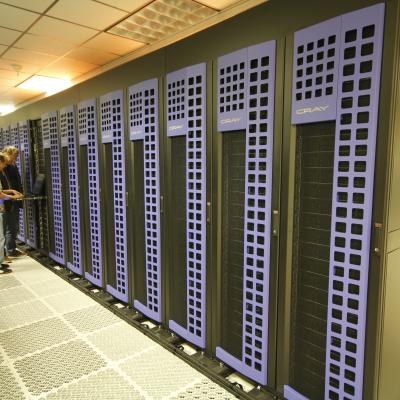
Clinical images have a wealth of data that are currently untapped by physicians and machine learning (ML) methods alike. Most ML methods require more data than is available to sufficiently train them. In order to obtain all data contained in a clinical image, it is imperative to be able to utilize multimodal, or various types of, data such as tags or identifications, especially where spatial…
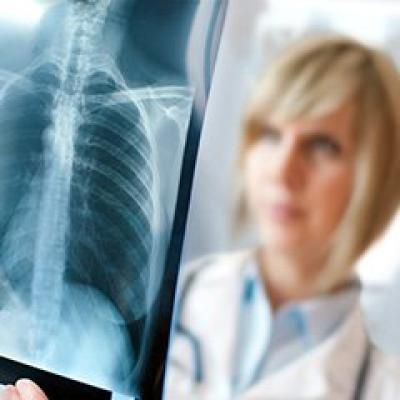
Some COVID-19 diagnoses are utilizing computed tomography (CT)-scans for triage. CT-scans produce immediate results with high sensitivity. The digital images produced by a CT-scan require physicians to identify objects within the image to determine the presence of disease. Object identification can be done using machine learning (ML) techniques such as deep learning (DL) to improve speed and…
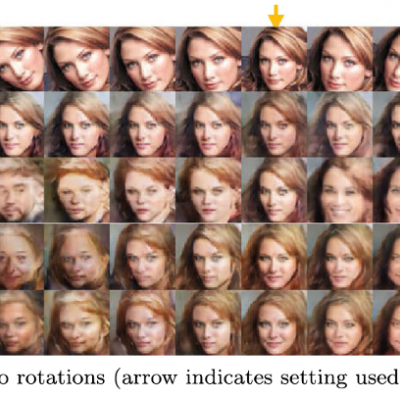
MimicGAN represents a new generation of methods that can “self-correct” for unseen corruptions in the data out in the field. This is particularly useful for systems that need to be deployed autonomously without needing constant intervention such as Automated Driver Assistance Systems. MimicGAN achieves this by treating every test sample as “corrupt” by default. The goal is to determine (a) the…
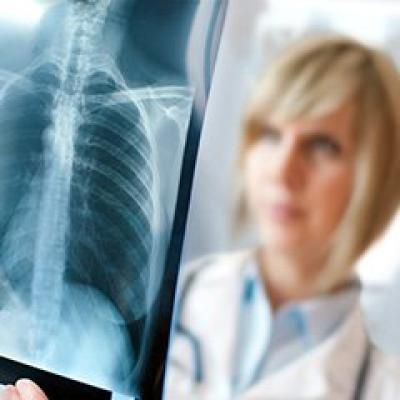
LLNL has developed a new system, called the Segmentation Ensembles System, that provides a simple and general way to fuse high-level and low-level information and leads to a substantial increase in overall performance of digital image analysis. LLNL researchers have demonstrated the effectiveness of the approach on applications ranging from automatic threat detection for airport security, to…