LLNL’s Distributed Implicit Neural Representation (DINR) is a novel approach to 4D time-space reconstruction of dynamic objects. DINR is the first technology to enable 4D imaging of dynamic objects at sufficiently high spatial and temporal resolutions that are necessary for real world medical and industrial applications.
Keywords
- Show all (234)
- Additive Manufacturing (53)
- Instrumentation (41)
- Synthesis and Processing (20)
- Sensors (14)
- Diagnostics (12)
- Imaging Systems (9)
- Photoconductive Semiconductor Switches (PCSS) (9)
- 3D Printing (8)
- Electric Grid (7)
- Materials for Energy Products (7)
- Substrate Engraved Meta-Surface (SEMS) (7)
- Therapeutics (7)
- Carbon Utilization (6)
- Semiconductors (6)
- Brain Computer Interface (BCI) (5)
- Compact Space Telescopes (5)
- Data Science (5)
- Optical Switches (5)
- Diode Lasers (4)
- (-) Laser Materials Processing (4)
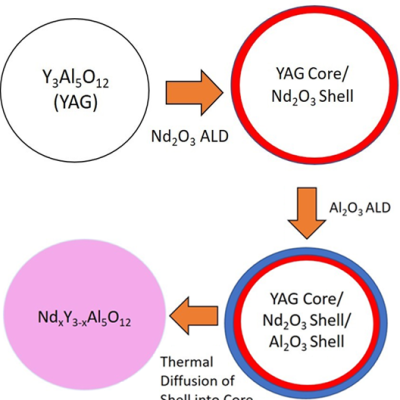
Powder atomic layer deposition process is used to coat nanopowders of host materials (e.g. yttrium aluminum garnet) with optically active neodymium organometal precursor followed by O2/O3 RF plasma to convert to a single layer of Nd2O3. The process can be repeated to build arbitrarily thick layers with custom doping profiles and followed by post-…
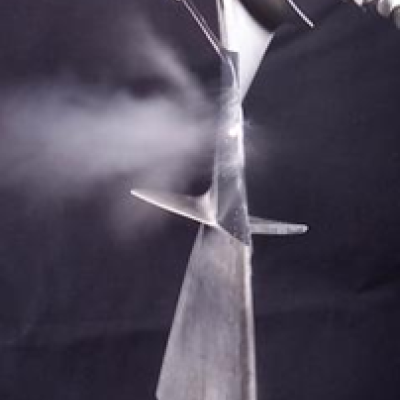
This invention proposes using a pulse laser configured to generate laser pulses and a controller for controlling operation of the pulse laser. The controller is further configured to control the pulse laser to cause the pulse laser to generate at least one of the laser pulses with a spatiotemporally varying laser fluence over a duration of at least one of the laser pulses. The spatiotemporally…
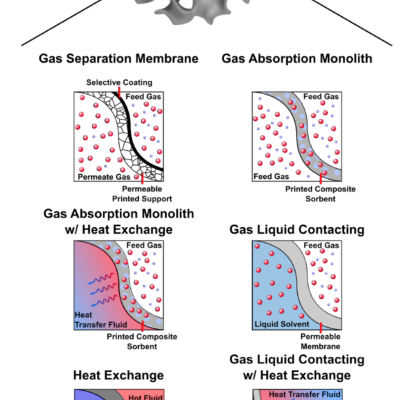
LLNL researchers have devised a set of design principles that facilitates the development of practical TPMS-based two fluid flow reactors.; included in the design are these new concepts:
![Filled (8,8) (left) and (15,15) (right) CNTs with [EMIM+][BF4- ] using SGTI with the proposed spliced soft-core potential (SSCP) approach](/sites/default/files/styles/scale_exact_400x400_/public/2023-10/Filled%20CNTs%20using%20SGTI.png?itok=Dy0ObN7i)
LLNL researchers have developed a novel simulation methodology using slow growth thermodynamic integration (SGTI) utilizing spliced soft-core interaction potential (SSCP). The approach to filling the molecular enclosures is a nonphysical one. Rather than filling the pores from the open ends this method creates steps in the algorithm that allow molecules to pass through the pore…
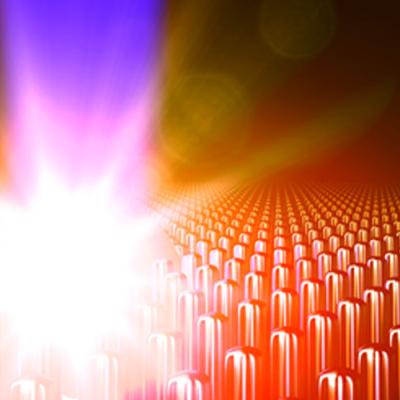
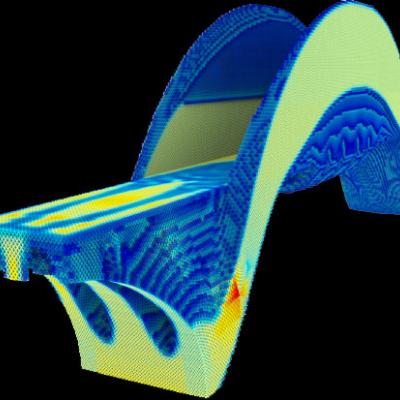
The LiDO code combines finite element analysis, design sensitivity analysis and nonlinear programming in a High-Performance Computing (HPC) environment that enables the solution of large-scale structural optimization problems in a computationally efficient manner. Currently, the code uses topology optimization strategies in which a given material is optimally distributed throughout the domain…
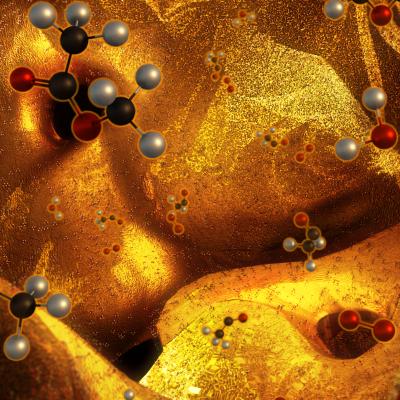
By combining 3D printing and dealloying., researchers at LLNL have developed a method for fabricating metal foams with engineered hierarchical architectures consisting of pores at least 3 distinct length scales. LLNL’s method uses direct ink writing (DIW), a 3D printing technique for additive manufacturing to fabricate hierarchical nanoporous metal foams with deterministically controlled 3D…
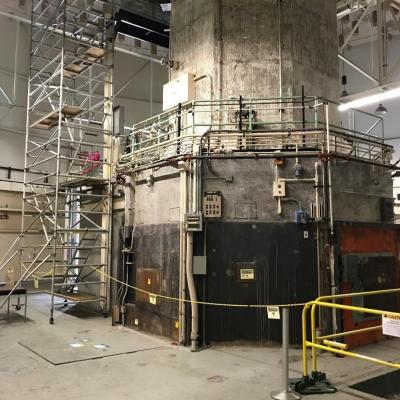
The new LLNL technique works by transiently removing and trapping concrete or rock surface material, so that contaminants are confined in a manner that is easy to isolate and remove. Our studies suggest that 10 m2 of surface could be processed per hour. The technique easily scales to more surface/hr.
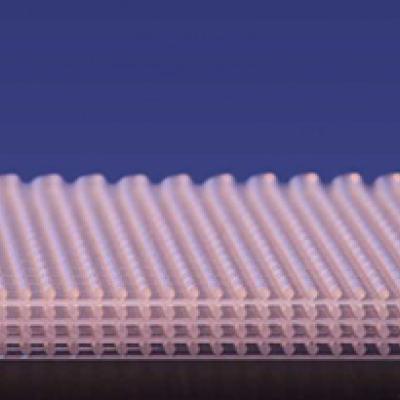
To overcome limitations with cellular silicone foams, LLNL innovators have developed a new 3D energy absorbing material with tailored/engineered bulk-scale properties. The energy absorbing material has 3D patterned architectures specially designed for specific energy absorbing properties. The combination of LLNL's capabilities in advanced modeling and simulation and the additive…