For this method, a Silicon on Insulator (SOI) wafer is used to tailor etch rates and thickness in initial steps of the process. The simple three step process approach is comprised of grayscale lithography, deep reactive-ion etch (DRIE) and liftoff of the SOI wafer. The liftoff process is used to dissolve the insulating layer, thus separating sections of the wafer as individual silicon…
Keywords
- Show all (101)
- Additive Manufacturing (37)
- Photoconductive Semiconductor Switches (PCSS) (9)
- Imaging Systems (8)
- 3D Printing (7)
- Semiconductors (6)
- Optical Switches (4)
- Electric Grid (3)
- Manufacturing Improvements (3)
- Power Electronics (3)
- Sensors (3)
- Computing (2)
- Optical Sensors (2)
- Particle Accelerators (2)
- Spectrometers (2)
- Synthesis and Processing (2)
- Manufacturing Simulation (1)
- Volumetric Additive Manufacturing (1)
- (-) Manufacturing Automation (2)
- (-) MEMS Sensors (2)
- (-) Precision Engineering (2)
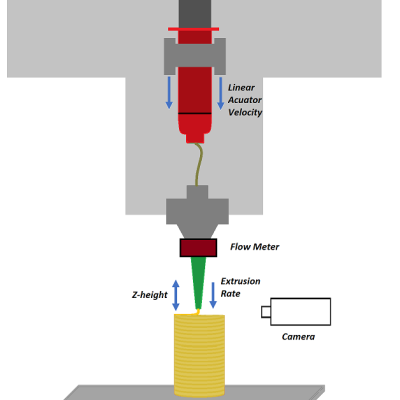
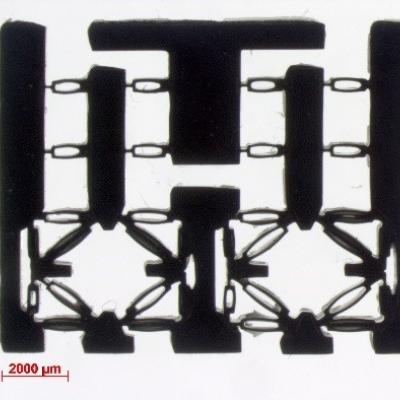
Recent advancements in additive manufacturing, also called 3D printing, allow precise placement of materials in three dimensions. LLNL researchers have invented mechanical logic gates based on flexures that can be integrated into the microstructure of a micro-architected material through 3D printing. The logic gates can be combined into circuits allowing complex logic operations to be…
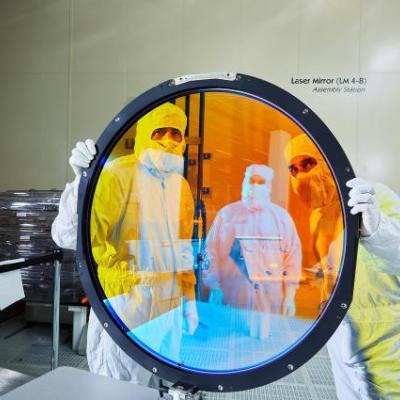
The LLNL method for optimizing as built optical designs uses insights from perturbed optical system theory and reformulates perturbation of optical performance in terms of double Zernikes, which can be calculated analytically rather than by tracing thousands of rays. A new theory of compensation is enabled by the use of double Zernikes which allows the performance degradation of a perturbed…
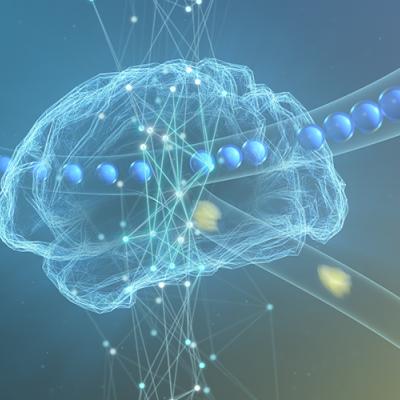
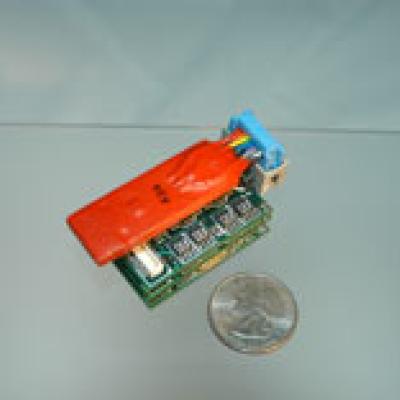
LLNL has developed a compact and low-power cantilever-based sensor array, which has been used to detect various vapor-phase analytes. For further information on the latest developments, see the article "Sniffing the Air with an Electronic Nose."