LLNL researchers have developed a method to enhance the performance of polyelectrolyte membranes by using a humidity-controlled crosslinking process which can be applied to precisely adjust the water channels of the membrane.
Keywords
- Show all (114)
- Additive Manufacturing (53)
- Synthesis and Processing (20)
- 3D Printing (8)
- Materials for Energy Products (6)
- Material Design (4)
- Microfabrication (3)
- Additively Manufactured (AM) Optics (2)
- Manufacturing Improvements (2)
- Rare Earth Elements (REEs) (2)
- Volumetric Additive Manufacturing (2)
- Direct Air Capture (1)
- Electric Grid (1)
- Inertial Confinement Fusion (ICF) (1)
- Inertial Fusion Energy (IFE) (1)
- Magnet Compositions (1)
- Manufacturing Simulation (1)
- Material Characterization (1)
- Precision Engineering (1)
- (-) Manufacturing Automation (2)
- (-) Membranes (2)
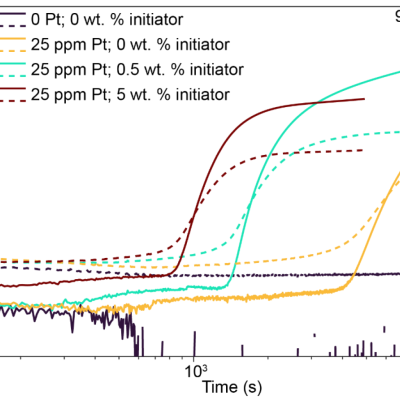
The approach is to use peroxides to modify the reaction kinetics in the production of polysiloxanes. A radical initiator in the presence of a hydride-terminated polysiloxane will increase the rate of curing and reduce manufacturing costs. At a minimum a formulation would contain a hydride-terminated polysiloxane, a platinum catalyst, and an initiator that generates radicals. …
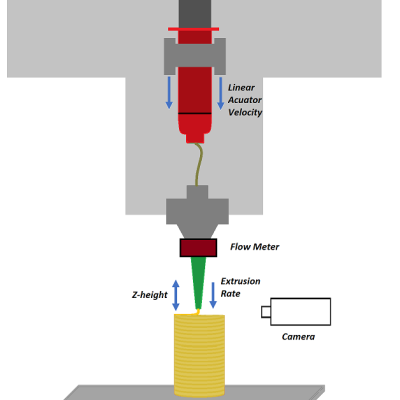
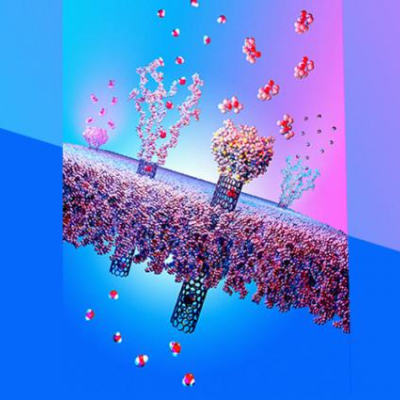
LLNL researchers have developed an alternative route to protective breathable membranes called Second Skin technology, which has transformative potential for protective garments. These membranes are expected to be particularly effective in mitigating physiological burden.
For additional information see article in Advanced Materials “Ultrabreathable and Protective Membranes with Sub-5…
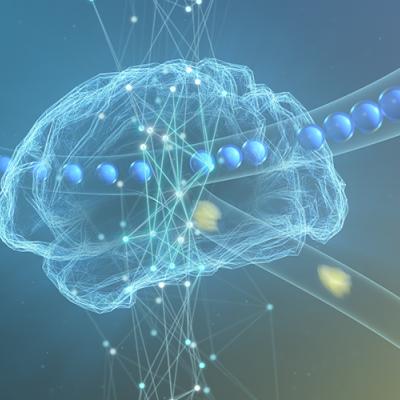