LLNL researchers have developed a TDLAS-based, standalone, real-time gas analyzer in a small form-factor for continuous or single-point monitoring. The system can analyze multiple gases with ultra-high sensitivity (ppm detection levels) in harsh conditions when utilizing wavelength-modulation spectroscopy (WMS).
Keywords
- Show all (101)
- Additive Manufacturing (37)
- Photoconductive Semiconductor Switches (PCSS) (9)
- Imaging Systems (8)
- 3D Printing (7)
- Semiconductors (6)
- Optical Switches (4)
- Electric Grid (3)
- Manufacturing Improvements (3)
- Power Electronics (3)
- Computing (2)
- MEMS Sensors (2)
- Optical Sensors (2)
- Particle Accelerators (2)
- Precision Engineering (2)
- Spectrometers (2)
- Synthesis and Processing (2)
- Manufacturing Simulation (1)
- Volumetric Additive Manufacturing (1)
- (-) Sensors (3)
- (-) Manufacturing Automation (2)
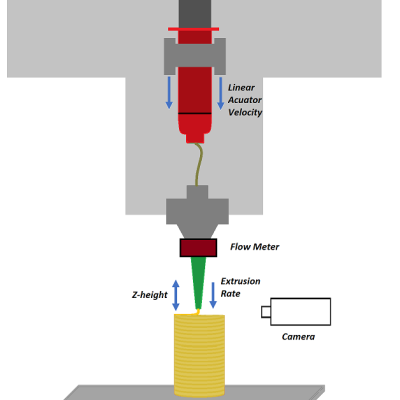
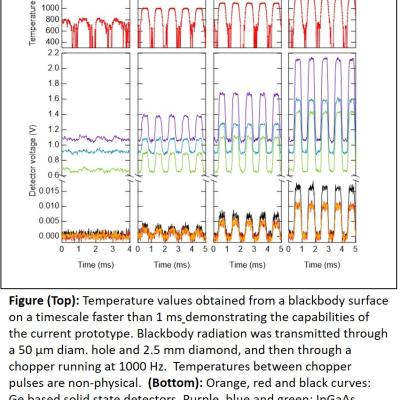
LLNL researchers have designed and tested performance characteristics for a multichannel pyrometer that works in the NIR from 1200 to 2000 nm. A single datapoint without averaging can be acquired in 14 microseconds (sampling rate of 70,000/s). In conjunction with a diamond anvil cell, the system still works down to about 830K.
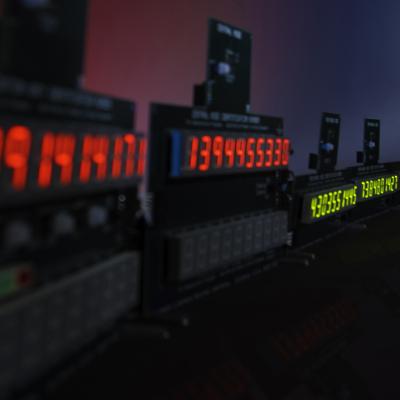
LLNL's method of equivalent time sampling incorporates an embedded system that generates the pulses used to trigger the external circuit and the data acquisition (DAQ). This removes the external reference clock, allowing the overall system clock rate to change based on the ability of the embedded system. The time delays needed to create the time stepping for equivalent time sampling is done by…
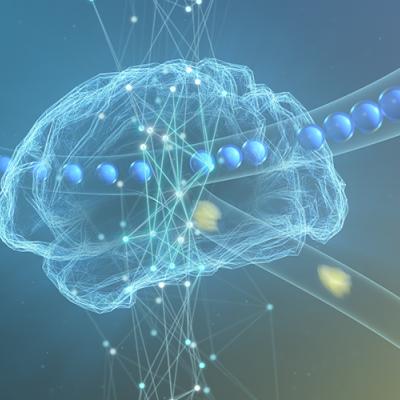
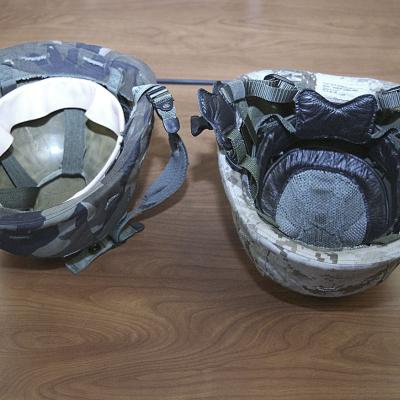
LLNL's high fidelity hydrocode is capable of predicting blast loads and directly coupling those loads to structures to predict a mechanical response. By combining this code and our expertise in modeling blast-structure interaction and damage, along with our access to experimental data and testing facilities, we can contribute to the design of protective equipment that can better mitigate the…