LLNL’s high throughput method involves proteome-wide screening for linear B-cell epitopes using native proteomes isolated from a pathogen of interest and convalescent sera from immunized animals. LLNL researchers have applied their newly developed generalizable screening method to the identification of pathogenic bacteria by screening linear B-cell epitopes in the proteome of Francisella…
Keywords
- Show all (143)
- Instrumentation (38)
- Additive Manufacturing (37)
- Sensors (16)
- Diagnostics (13)
- 3D Printing (7)
- Therapeutics (5)
- Ground Penetrating Radar (4)
- Brain Computer Interface (BCI) (3)
- Defense Technologies (3)
- Manufacturing Improvements (3)
- National Security Forensics (2)
- Synthesis and Processing (2)
- Electric Grid (1)
- Manufacturing Simulation (1)
- Material Design (1)
- Microfabrication (1)
- Precision Engineering (1)
- Volumetric Additive Manufacturing (1)
- (-) Manufacturing Automation (2)
- (-) Vaccines (2)
Technology Portfolios
Image
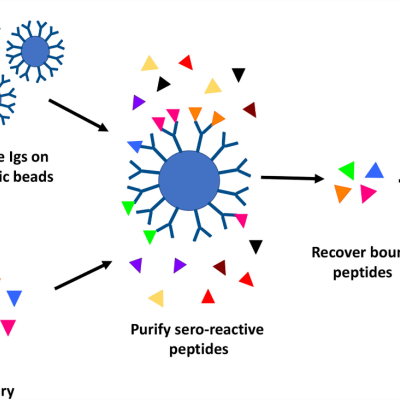
High throughput discovery of linear B-cell epitopes for the development of vaccines and therapeutics
Image
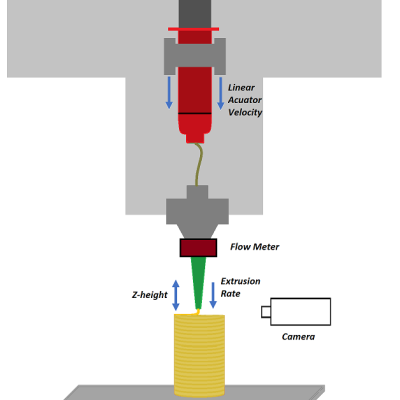
Livermore researchers have developed a method for implementing closed-loop control in extrusion printing processes by means of novel sensing, machine learning, and optimal control algorithms for the optimization of printing parameters and controllability. The system includes a suite of sensors, including cameras, voltage and current meters, scales, etc., that provide in-situ process monitoring…
Image
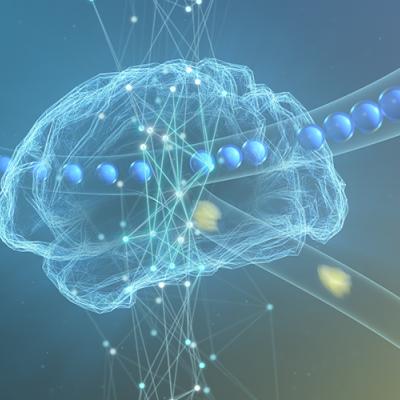
LLNL researchers have developed a system that relies on machine learning to monitor microfluidic devices. The system includes (at least) a microfluidic device, sensor(s), and a local network computer. The system could also include a camera that takes real-time images of channel(s) within an operating microfluidic device. A subset of these images can be used to train/teach a machine learning…
Image
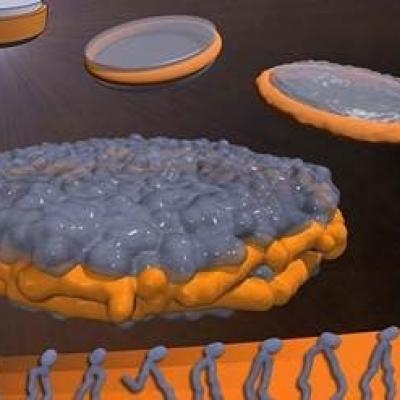
LLNL has developed a novel process of production, isolation, characterization, and functional re-constitution of membrane-associated proteins in a single step. In addition, LLNL has developed a colorimetric assay that indicates production, correct folding, and incorporation of bR into soluble nanolipoprotein particles (NLPs).
LLNL has developed an approach, for formation of NLP/…