A set of images generated by multiple passes over the same area can be coherently integrated by this technology developed by LLNL researchers. The primary difficulty with coherently combining different passes is registering the images obtained from each pass, particularly if a pass only partially covers a given area.
Keywords
- Show all (228)
- Additive Manufacturing (51)
- Instrumentation (40)
- Synthesis and Processing (19)
- Sensors (14)
- Diagnostics (12)
- Imaging Systems (9)
- Photoconductive Semiconductor Switches (PCSS) (9)
- 3D Printing (7)
- Electric Grid (7)
- Materials for Energy Products (7)
- Substrate Engraved Meta-Surface (SEMS) (7)
- Therapeutics (7)
- Carbon Utilization (6)
- Semiconductors (6)
- Compact Space Telescopes (5)
- Optical Switches (5)
- Diode Lasers (4)
- Laser Materials Processing (4)
- Precision Optical Finishing (4)
- (-) Data Science (5)
Technology Portfolios
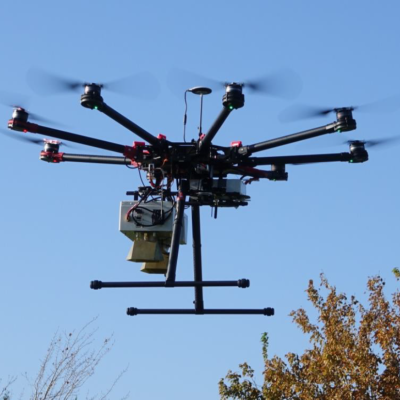
LLNL researchers have developed a lightweight drone-based GPR array that when flown over a surface with laid and/or buried objects could image the field of view and be able to detect targets and discriminate them from clutter. The imaging method employs a modified multi-static architecture to provide the highest signal to noise with the lowest system weight, making it ideal for airborne or…
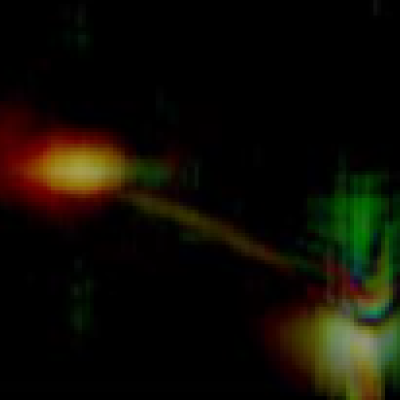
This technology uses three different frequency bands to create intensity maps of returned signals. Signals have traditionally been displayed as raw return data. The intensity of the return is represented by level of brightness. Assignment of a scalar value for intensity is used to determine the brightness of the image. In this technology, each frequency is given a designated…
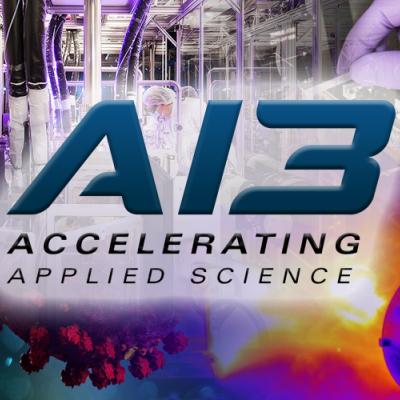
Lawrence Livermore National Laboratory (LLNL) is offering the opportunity to collaborate in accelerating artificial intelligence (AI) for applied science, including research in key areas such as advanced material design, 3D printing, predictive biology, energy systems, “self-driving” lasers and fusion energy research.
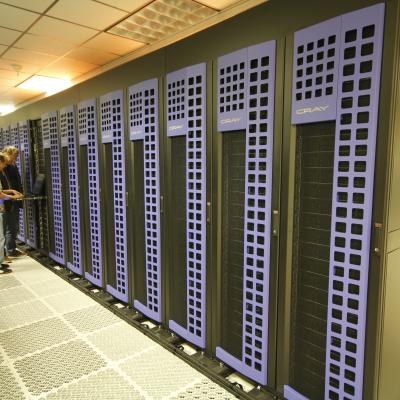
Clinical images have a wealth of data that are currently untapped by physicians and machine learning (ML) methods alike. Most ML methods require more data than is available to sufficiently train them. In order to obtain all data contained in a clinical image, it is imperative to be able to utilize multimodal, or various types of, data such as tags or identifications, especially where spatial…
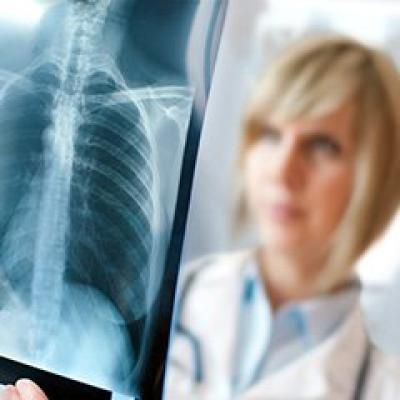
Some COVID-19 diagnoses are utilizing computed tomography (CT)-scans for triage. CT-scans produce immediate results with high sensitivity. The digital images produced by a CT-scan require physicians to identify objects within the image to determine the presence of disease. Object identification can be done using machine learning (ML) techniques such as deep learning (DL) to improve speed and…
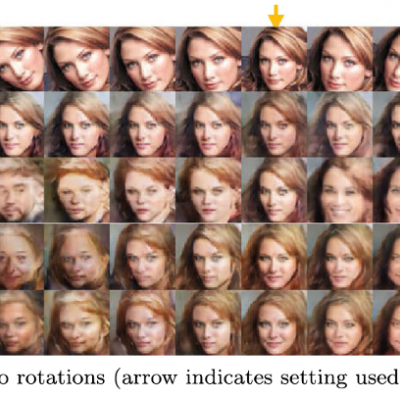
MimicGAN represents a new generation of methods that can “self-correct” for unseen corruptions in the data out in the field. This is particularly useful for systems that need to be deployed autonomously without needing constant intervention such as Automated Driver Assistance Systems. MimicGAN achieves this by treating every test sample as “corrupt” by default. The goal is to determine (a) the…
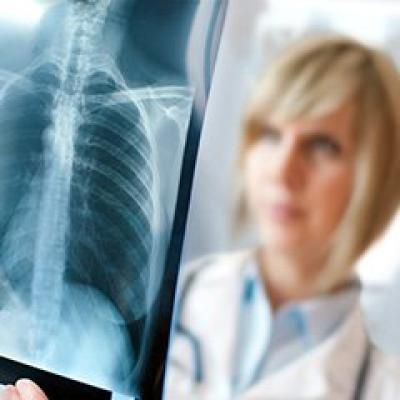
LLNL has developed a new system, called the Segmentation Ensembles System, that provides a simple and general way to fuse high-level and low-level information and leads to a substantial increase in overall performance of digital image analysis. LLNL researchers have demonstrated the effectiveness of the approach on applications ranging from automatic threat detection for airport security, to…
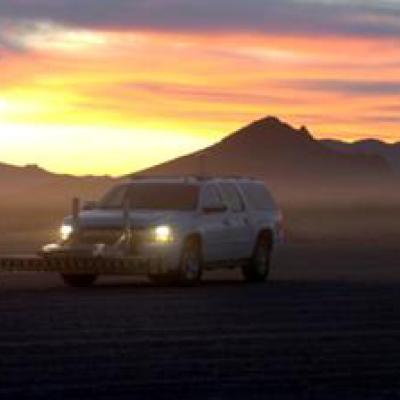
LLNL has developed a wide band (WB) ground penetrating radar (GPR) technology to detect and image buried objects under a moving vehicle. Efficient and high performance processing algorithms reconstruct images of buried or hidden objects in two or three dimensions under a scanning array. The technology includes a mobile high-performance computing system allowing GPR array sensor data to be…