LLNL’s novel approach utilizes a number of techniques to improve reconstruction accuracy:
Image
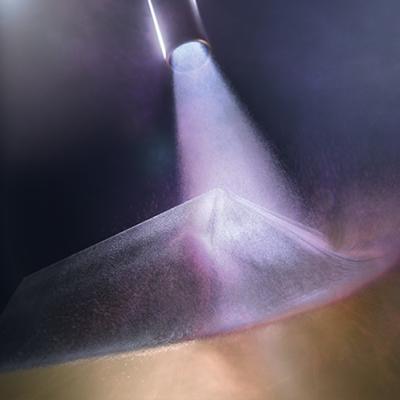
Versatile Cold Spray (VCS) enables deposition of brittle materials, such as thermoelectrics, magnets, and insulators, while retaining their functional properties. Materials can be deposited on substrates or arbitrary shapes with no requirement to match compositions. The VCS system is low cost, easily portable, and easy to use.
VCS has been developed in a collaboration between Lawrence Livermore…
Image
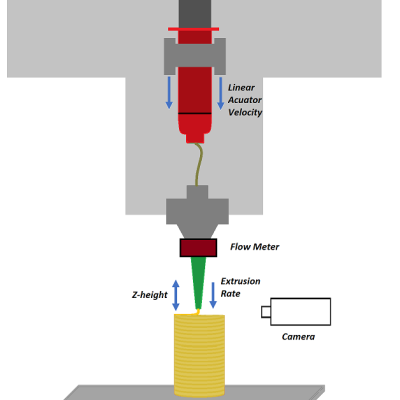
Livermore researchers have developed a method for implementing closed-loop control in extrusion printing processes by means of novel sensing, machine learning, and optimal control algorithms for the optimization of printing parameters and controllability. The system includes a suite of sensors, including cameras, voltage and current meters, scales, etc., that provide in-situ process monitoring…
Image
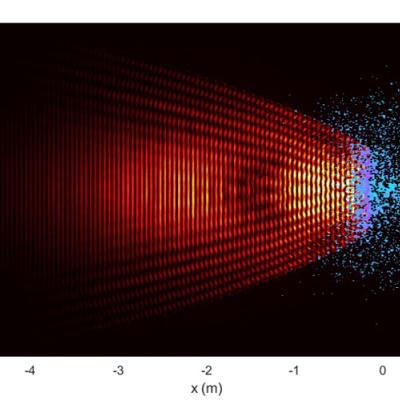
LLNL pioneered the use of tomographic reconstruction to determine the power density of electron beams using profiles of the beam taken at a number of angles. LLNL’s earlier diagnostic consisted of a fixed number of radially oriented sensor slits and required the beam to be circled over them at a fixed known diameter to collect data. The new sensor design incorporates annular slits instead,…
Image
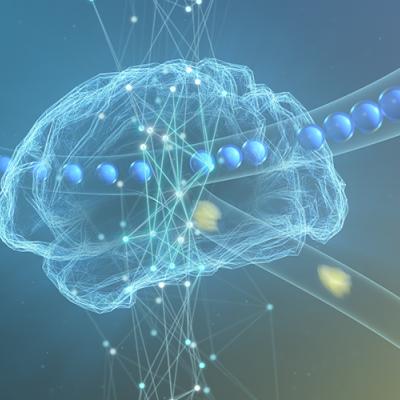
LLNL researchers have developed a system that relies on machine learning to monitor microfluidic devices. The system includes (at least) a microfluidic device, sensor(s), and a local network computer. The system could also include a camera that takes real-time images of channel(s) within an operating microfluidic device. A subset of these images can be used to train/teach a machine learning…